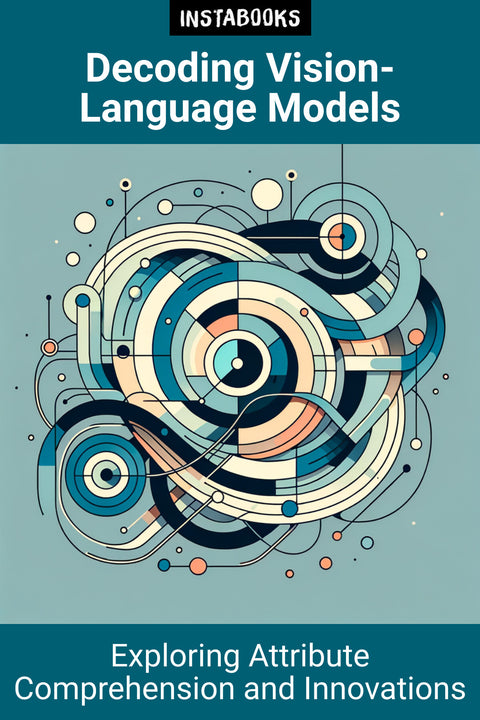
Instabooks AI (AI Author)
Decoding Vision-Language Models
Exploring Attribute Comprehension and Innovations
Premium AI Book (PDF/ePub) - 200+ pages
Introduction to Vision-Language Models
In the rapidly evolving field of artificial intelligence, large vision-language models (LVLMs) have emerged as groundbreaking tools capable of transforming how machines perceive and interpret visual data. A comprehensive evaluation of these models, focusing specifically on attribute comprehension, is critical to unlocking their full potential. This book delves into both theoretical aspects and practical applications, providing a robust framework for understanding how these models operate, the challenges they face, and the groundbreaking methods designed to enhance their capabilities.
Innovations in Attribute Comprehension
Attribute comprehension serves as a cornerstone for LVLMs, demanding a nuanced understanding of visual attributes. The book explores recent advancements, such as the PRIS-CV benchmark and the revolutionary ArGue method, illustrating how these tools and methodologies refine the models' abilities to recognize and generate attributes. Through detailed case studies and analyses, readers gain insights into the intricate processes of visual question answering, image-text matching, and more, emphasizing how these interactions enhance model proficiency.
Advancing Attribute-Guided Prompt Tuning
Attribute-guided prompt tuning represents a critical stride in refining model performance. This book presents a deep dive into techniques that leverage attribute information to guide prompt tuning, boosting LVLM efficacy. With practical examples and scenarios, the book illustrates the transformative impact of prompt tuning on specialized and general tasks, underscoring its importance for future research and development.
Challenges and Solutions in Multi-Modal Understanding
Though LVLMs present immense possibilities, they also face significant challenges in multi-modal understanding. This book offers an in-depth analysis of issues such as object hallucination and text-image interference, paving the way for innovative solutions. By understanding these hurdles and the current research efforts aimed at overcoming them, readers can appreciate the strides being made to enhance model robustness and cognitive capabilities.
The Future of LVLMs and Cognitive Advancement
As ongoing research efforts continue to evolve, the future landscape of LVLMs looks promising. This book highlights current trends and potential future directions, fostering a culture of innovation and inquiry. By enhancing the robustness of attribute comprehension, researchers and practitioners alike can significantly improve the models' application across various domains. This book is a critical resource for anyone seeking to understand and contribute to the advancement of vision-language models.
Table of Contents
1. Introduction to Vision-Language Models- Foundations of LVLMs
- Historical Context and Evolution
- Importance in Modern AI
2. Evaluating Attribute Comprehension
- Core Concepts and Metrics
- PRIS-CV Benchmark Analysis
- Case Studies and Applications
3. The ArGue Method: An Overview
- Introduction to ArGue
- Techniques for Attribute Recognition
- Impact and Innovations
4. Attribute-Guided Prompt Tuning
- Techniques and Strategies
- Enhancing Model Performance
- Examples and Outcomes
5. Performance in Specialized Tasks
- Challenges in Specialized Domains
- Evaluation of Model Capabilities
- Case Studies in Specialized Tasks
6. Performance in General Tasks
- Understanding General Task Dynamics
- Model Strengths and Weaknesses
- Future Directions in General Task Evaluation
7. Challenges in Multi-Modal Understanding
- Object Hallucination Issues
- Image-Text Interference
- Overcoming Limitations
8. Recent Advancements and Techniques
- Innovations in LVLMs
- Comparative Studies
- Impact on Field Evolution
9. Current Research and Developments
- State of the Art in LVLMs
- Collaborative Efforts
- Future Research Directions
10. Enhancing Robustness in LVLMs
- Techniques for Greater Cognitive Power
- Robustness in Complex Scenarios
- Impact of High-Quality Data
11. The Future of Vision-Language Models
- Predictive Trends
- Technological Innovations
- Transformation in Multimodal AI
12. Conclusion and Outlook
- Summarizing Key Insights
- Strategic Outlook for LVLMs
- Encouraging Future Inquiry
Target Audience
This book is designed for AI researchers, data scientists, and professionals interested in the development of vision-language models and attribute comprehension.
Key Takeaways
- Understand the core principles and advancements in vision-language models (LVLMs).
- Explore the innovative ArGue method for enhancing attribute comprehension.
- Gain insights into attribute-guided prompt tuning for improved model performance.
- Analyze challenges in multi-modal understanding and potential solutions.
- Discover the future trends and ongoing research efforts shaping LVLMs.
How This Book Was Generated
This book is the result of our advanced AI text generator, meticulously crafted to deliver not just information but meaningful insights. By leveraging our AI book generator, cutting-edge models, and real-time research, we ensure each page reflects the most current and reliable knowledge. Our AI processes vast data with unmatched precision, producing over 200 pages of coherent, authoritative content. This isn’t just a collection of facts—it’s a thoughtfully crafted narrative, shaped by our technology, that engages the mind and resonates with the reader, offering a deep, trustworthy exploration of the subject.
Satisfaction Guaranteed: Try It Risk-Free
We invite you to try it out for yourself, backed by our no-questions-asked money-back guarantee. If you're not completely satisfied, we'll refund your purchase—no strings attached.